MariaDB Vector and RAG at foss-north 2025
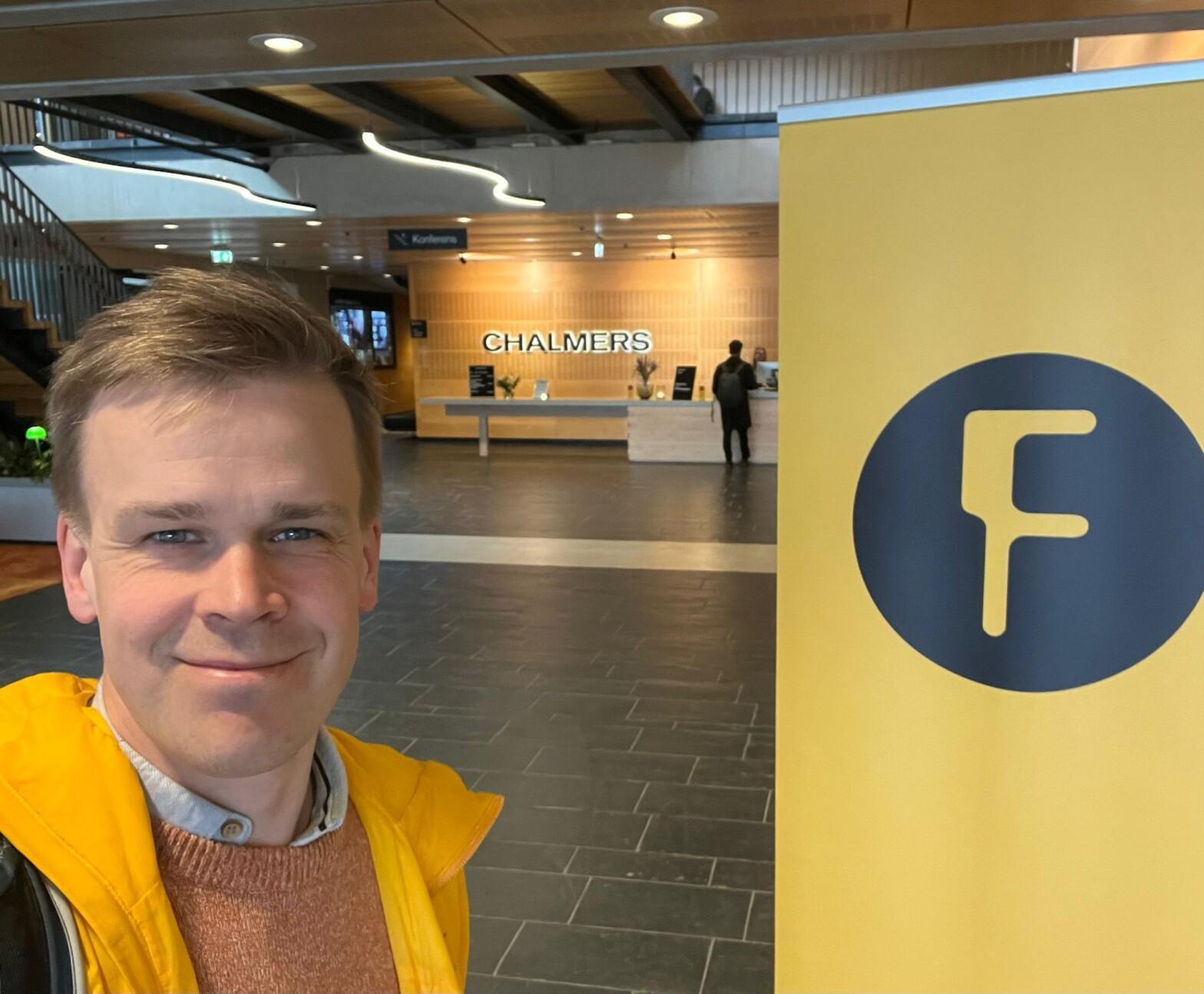
MariaDB Vector part of MariaDB 11.8 LTS. See the latest about vector on the MariaDB Vector project page.
I had the delight of being accepted to talk about “Making AI transparent with RAG on your own data” at foss-north 2025 in Gothenburg, Sweden. On April 14th at Chalmers University, I got to share with a full room on how AI RAG is done with MariaDB Vector, kicking off with a use case about scaling Wikipedia editing with GenAI.
Improving Wikipedia with RAG
I presented a use case example from my experience of editing Wikipedia at scale with GenAI for non-profit Projekt Fredrika. A bottleneck when trying to edit Wikipedia at scale, is finding all relevant Wikipedia pages that can be improved with a certain source. RAG can help in both matching sources with suitable Wikipedia articles, and generating AI improvements with source references – a crucial requirement for any additions to Wikipedia. All in all, a suitable challenge for RAG.
Doing RAG with MariaDB Vector
I then went through the technical aspects of doing RAG with MariaDB Vector. I explained the benefits of storing vectors in the same database with your data, instead of a dedicated vector database.
I’ll recap the steps in short – for more details see Try RAG with MariaDB Vector on your own MariaDB data!.
- In a preparation phase, the source text is cut into suitable text parts, “chunks”. Chunks are transformed into vectors with an embedding model and inserted into MariaDB Vector.
- At run-time, the first step is to vectorize the user text prompt, and do a vector search to find what chunks of information are most relevant to answer the question. The second step is to combine the questions and the context chunks in a prompt, and give it to a Large Language Model to generate the actual answer to the question – using only the relevant chunks that were fetched.
Audience questions
I was curious about my audience. A show of hands showed they were in general tech savvy, but less familiar with AI tech. Among databases they were familiar with MySQL, Postgres, slightly less with MariaDB, and nearly not at all with dedicated vector storages. Only a couple of people had tried RAG, or more complex LLM prompts before – which is perhaps expected for people coming to learn about this subject.
There were two questions from the audience, which I thought I’d rephrase in this blog:
- What are the best strategies for chunkification? It depends on the material. If you are searching among many short documents, it might be enough to vectorize the title or introduction of a document to find the document you need. In other cases you want to break the document down into smaller chunks to find the right chapter, page, paragraph or even sentence within it. You need to try out a couple of approaches to see which one suits your use case.
- MariaDB uses nearest neighbour search – what about others such as BM25? BM25 is a page ranking method for search engines which is currently not on the MariaDB roadmap. MariaDB makes combining vector search with basic SQL filtering possible which can be beneficial for RAG solutions. Other search options could be e.g. hybrid search that combines vector and keyword search.
Foss-north in general
MariaDB’s Vector and RAG talk was preceded and complemented by Red Hat’s workshop on AI fine-tuning – an alternative approach to adapting Generative AI to your needs.
Foss-north was kicked off by the creator of cURL, Daniel Stenberg from Sweden, talking about the general situation of open source. He raised questions such as the popularity of open source, maintenance of projects, the rising average age of open source contributors, concerns over project independence in a turbulent world, and use of AI in open source development – he is not a fan due to negative implications, though as an optimist, I think it’s benefits will surpass its drawbacks.
All in all a great event with interesting talks. Many thanks to the organizers!
See also
- The presentation’s recording on Youtube (43:10): Making AI transparent with RAG on your own data
- MariaDB Vector: https://mariadb.com/kb/en/vector-overview/
- RAG with MariaDB Vector: https://mariadb.org/rag-with-mariadb-vector/